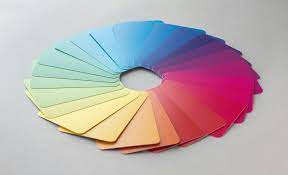
Color prediction gaming has gained significant popularity due to its simplicity, instant gratification, and potential rewards. While many players rely on luck to make their predictions, using statistical models can improve accuracy and enhance the overall gaming experience. By applying probability theory, pattern analysis, and predictive algorithms, players can make more informed decisions, increasing their chances of winning.
Understanding Probability in Color Prediction Gaming
At its core, color prediction gaming at 91 Club game involves selecting the correct color in a random event. This randomness can often be misleading, leading players to believe that previous results influence future outcomes. However, statistical models provide tools to analyze trends rather than rely on intuition alone.
Probability Distribution
Probability theory suggests that each color has an equal chance of appearing if the game is truly random. However, in many prediction games, there may be slight biases due to the system’s underlying mechanics.
- Uniform distribution: If a game has five colors, each color ideally has a 20% chance of appearing.
- Conditional probability: If past results indicate a pattern, conditional probability helps calculate the likelihood of a specific outcome based on prior data.
Players using probability models analyze historical data to determine if any colors appear more frequently than expected, refining their prediction strategy accordingly.
Applying Statistical Models for Prediction
Several statistical models can be used to enhance color prediction gaming strategies.
1. Bayesian Probability Model
The Bayesian model uses prior probabilities and adjusts them based on observed outcomes. Players can track color occurrences over multiple rounds and refine predictions based on frequency patterns. This model helps reduce randomness by continuously learning from past results, making predictions more accurate.
2. Markov Chains
The Markov Chain Model is useful when analyzing sequences in color prediction. It examines the probability of transitioning from one color to another based on previous rounds. By studying transition states, players can forecast the likelihood of a particular color appearing next, optimizing their prediction strategy.
For example, if the last four rounds followed a pattern like Red → Blue → Red → Blue, a Markov model can determine the probability of Red appearing again.
3. Regression Analysis
Regression models identify relationships between variables in prediction gaming. If external factors—such as time of day or previous betting patterns—affect color selections, regression analysis can uncover subtle influences that impact probabilities.
4. Monte Carlo Simulation
A Monte Carlo simulation generates thousands of random iterations to determine expected outcomes. Players can use this method to simulate multiple rounds, identify trends, and assess the likelihood of specific colors appearing within certain intervals.
Pattern Recognition and Machine Learning in Color Prediction
With advancements in AI, machine learning algorithms are transforming color prediction gaming strategies. Instead of manually calculating probabilities, automated models analyze real-time game data to forecast upcoming results.
1. Neural Networks and AI Prediction Models
Machine learning models, such as neural networks, use large datasets to recognize patterns and improve accuracy. AI-driven prediction models analyze thousands of game results, detecting subtle correlations that the human eye may miss.
2. Time-Series Analysis
Machine learning tools utilize time-series analysis to monitor trends in prediction games over time. If a pattern emerges—such as a particular color appearing more frequently at specific intervals—the system can flag the trend, helping players make strategic predictions.
Risk Management and Decision Optimization
While statistical models enhance predictions, they do not guarantee success. Players should use risk management techniques to maintain control over their gameplay:
- Setting Limits: Establishing loss limits prevents excessive spending based on false confidence in prediction models.
- Diversification: Instead of relying on a single strategy, players should incorporate multiple statistical models for balanced predictions.
- Avoiding Gambler’s Fallacy: Assuming that past results influence future outcomes incorrectly can lead to poor decision-making. Understanding true randomness is crucial.
Conclusion
Color prediction gaming is evolving from pure luck-based entertainment to a more data-driven experience. By integrating statistical models such as probability theory, Markov chains, Bayesian analysis, and machine learning techniques, players can refine their predictions and improve their outcomes. While no method guarantees success, using informed strategies increases the likelihood of accurate predictions, making the gaming experience both engaging and intellectually rewarding.
With AI and advanced analytics shaping the future of prediction gaming, players who embrace statistical models will gain a competitive edge, transforming casual play into strategic forecasting.
also read: Where to Find a Relaxing Brunch Restaurant in Dubai?